The Ultimate Guide to Data Labeling Tools: Unlocking the Power of Data Annotation
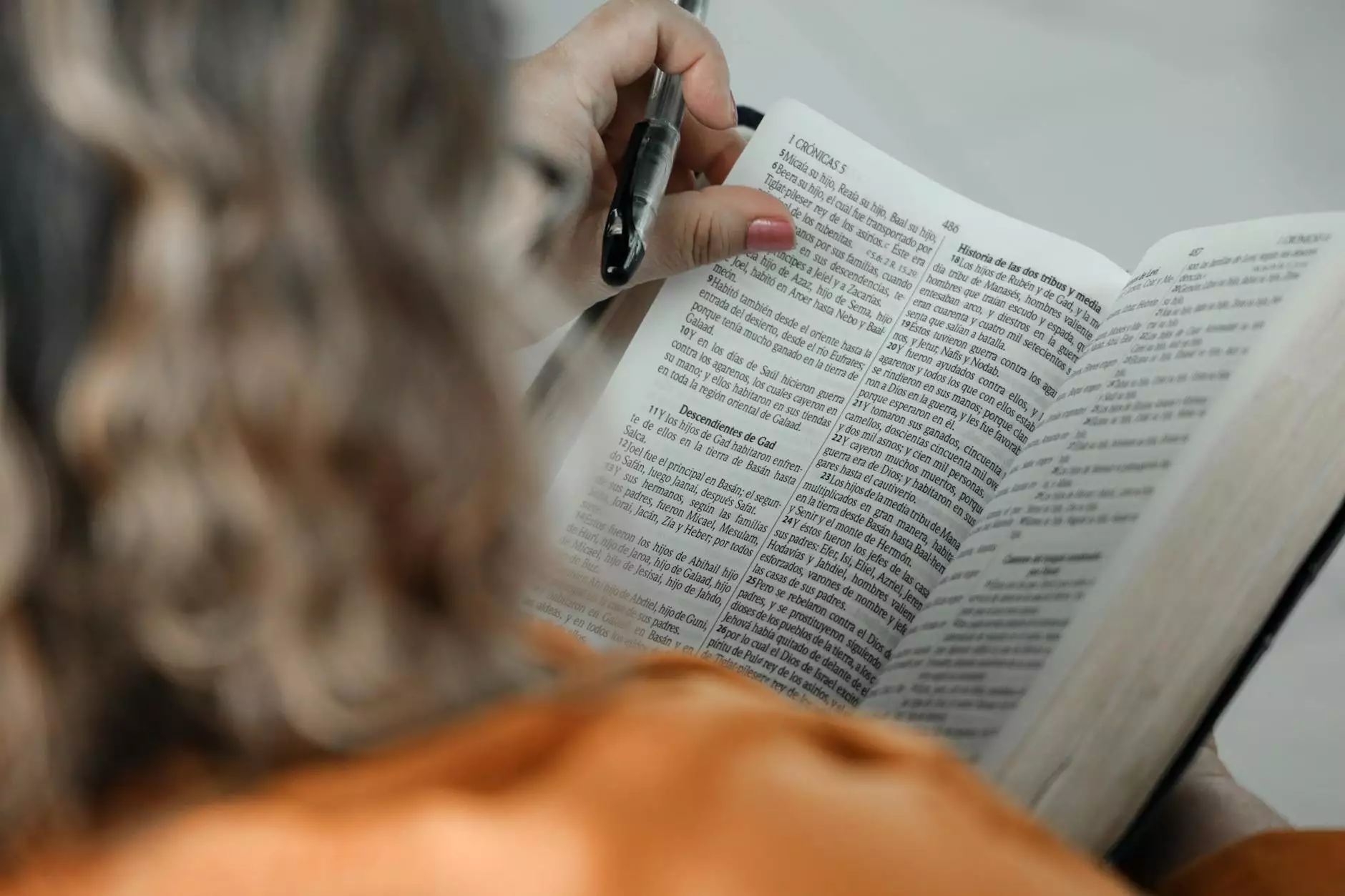
In today's digital age, the significance of data labeling tools cannot be understated. As artificial intelligence (AI) and machine learning (ML) continue to grow, the need for precise and efficient data annotation becomes vital. This comprehensive guide will delve into the world of data annotation platforms, illustrating their importance, benefits, and how they can transform the landscape of AI-driven projects.
What Are Data Labeling Tools?
Data labeling tools are software platforms designed to facilitate the process of annotating data. This process involves adding meaningful information to unstructured data, enabling machine learning algorithms to learn from this enriched dataset. The quality of the training data directly affects the performance of AI models, making these tools essential for any data-driven project.
Types of Data That Need Annotation
Various types of data require labeling, including:
- Images: Identifying objects, categorizing scenes, and recognizing features.
- Text: Sentiment analysis, entity recognition, and topic classification.
- Audio: Transcription, speaker identification, and emotion detection.
- Video: Object tracking, event recognition, and scene description.
Why Are Data Annotation Platforms Crucial for AI Development?
Data annotation platforms play a pivotal role in AI development by ensuring high-quality training datasets. Here are several reasons why they are essential:
1. Ensuring Accuracy
The accuracy of AI models is heavily reliant on the quality of labeled data. Data labeling tools streamline the annotation process, ensuring that each piece of data is accurately categorized and labeled, which leads to better performance of AI applications.
2. Scalability
As organizations scale their AI initiatives, the volume of data generated also increases. Robust data annotation platforms can handle large datasets efficiently, allowing businesses to annotate and utilize data at scale without sacrificing quality.
3. Speed and Efficiency
Manual data labeling can be a time-consuming task. Automation provided by data labeling tools accelerates the annotation process, allowing teams to focus on analyzing results rather than getting bogged down in data entry.
4. Cost-Effectiveness
By using data annotation platforms, organizations can minimize costs associated with manual labor and errors. With automated workflows and optimized processes, businesses can significantly lower their operational costs.
5. User-Friendly Interfaces
Many modern data labeling tools provide intuitive user interfaces that make it easy for users to label data without extensive training. This democratization of technology empowers teams across the organization, from data scientists to marketing professionals.
Choosing the Right Data Labeling Tool
With numerous data labeling tools available in the market, selecting the right one for your business can be overwhelming. Here are some critical factors to consider:
1. Annotation Types Supported
Ensure that the tool you select can handle the types of data you are working with, whether it be images, text, audio, or video. Some tools specialize in specific types, while others offer multi-modal capabilities.
2. Ease of Integration
Your data labeling tool should seamlessly integrate with your existing data pipelines and tools. Look for solutions that offer APIs and compatibility with popular data management systems.
3. Quality Assurance Features
A good data annotation platform should have built-in quality assurance mechanisms, such as review and feedback loops, to ensure that labeled data meets the necessary quality standards.
4. Scalability
As your data needs grow, your chosen tool should be able to scale accordingly. Whether you need to annotate thousands of images or millions of textual data points, ensure that the platform can handle your projected workload.
5. Support and Community
Reliable customer support and a strong user community can greatly enhance your experience with data labeling tools. Check for available resources, documentation, and forums to aid your team in utilizing the tool effectively.
Popular Data Labeling Tools to Consider
As you explore potential solutions for your data annotation needs, here are some recognized data labeling tools worth considering:
1. KeyLabs Data Annotation Platform
The KeyLabs Data Annotation Platform stands out for its versatility and user-friendly interface. It supports various data types, including images, text, and video, making it an ideal choice for businesses engaged in multiple AI projects. Moreover, KeyLabs emphasizes high-quality annotations and provides robust integration features with other tools.
2. Amazon SageMaker Ground Truth
Amazon SageMaker Ground Truth offers an efficient way to build highly accurate training datasets. Its machine learning capabilities aid in automating the data labeling process, thus reducing costs and time associated with manual labeling.
3. Labelbox
Labelbox is another notable tool that provides a comprehensive solution for data governance and collaboration. With its powerful annotation capabilities and workflow management features, teams can efficiently manage large annotation projects.
4. Supervisely
Supervisely caters specifically to computer vision tasks, making it an excellent option for teams working with image and video data. It boasts tools for annotation, dataset management, and model training in one integrated platform.
5. CVAT
The Computer Vision Annotation Tool (CVAT) is an open-source project developed by Intel. It is highly customizable and well-suited for teams with specific needs who want to maintain full control over their annotation process.
Best Practices for Using Data Labeling Tools
Maximizing the benefits of data labeling tools requires adherence to best practices that ensure efficiency and quality. Here are some recommendations:
1. Define Clear Annotation Guidelines
Develop comprehensive annotation guidelines that outline the standards and expectations for labeling data. This will help annotators maintain consistency and accuracy throughout the process.
2. Train Your Annotators
Invest time in training your annotators on the specifics of the task and the functionality of the data annotation platform. Well-trained annotators will produce higher quality annotations and reduce the need for rework.
3. Implement Quality Control Measures
Establish quality control processes such as random sampling, peer reviews, and iterative feedback to ensure the reliability of labeled data. Continuous evaluation can help catch and correct errors early in the process.
4. Utilize Collaborative Features
Most modern data labeling tools offer collaborative features that allow multiple team members to work together efficiently. Harness these capabilities to enhance productivity and streamline workflows.
5. Monitor Performance Metrics
Track the performance metrics of your data annotation projects. Analyze progress, quality, and time taken to improve future endeavors and refine your processes.
Conclusion: Harnessing the Power of Data Labeling Tools
In conclusion, the significance of data labeling tools in today's AI-driven landscape is immense. By investing in robust data annotation platforms, businesses can ensure high-quality training datasets, leading to the development of efficient and accurate AI models. As the demand for AI continues to rise, understanding and leveraging these tools is essential for organizations aiming to remain competitive in their respective fields.
Whether you choose to implement KeyLabs Data Annotation Platform or explore other available options, remember that quality data is the cornerstone of successful AI and ML initiatives. Embrace the advancements in data annotation and position your business at the forefront of technological innovation.